AI in The Workplace
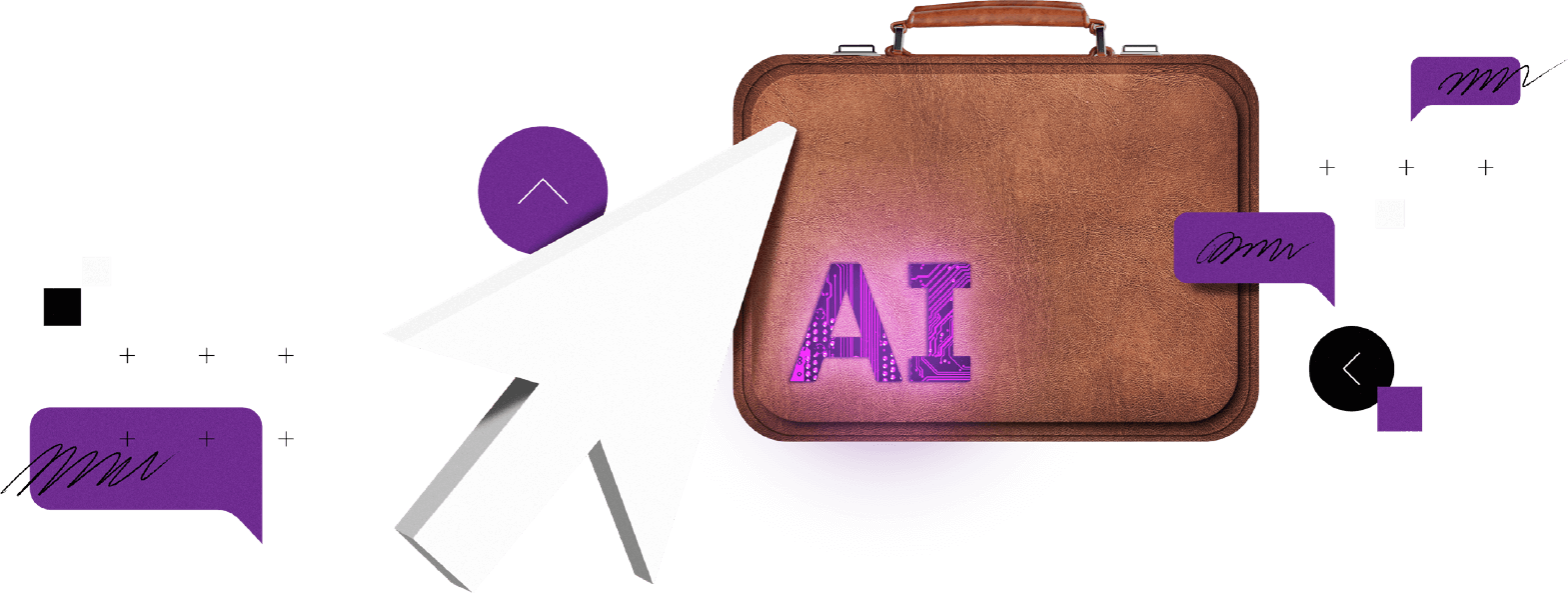
Dear readers,
On your screens a newsletter that has occupied us for a long time, a newsletter dedicated to an unprecedented technology, that feeds the imagination, fear and narratives about our own humanity.
In this issue we examine the present of the most talked-about intelligent technology worldwide, Artificial Intelligence, and offer insights and answers to key questions for the future of work and society, through articles written by specialized professionals and discussions with great experts and scientists.
What are the applications of AI in HR? How is the future of work changing with the advent of AI? What does data inclusion and debiasing of datasets mean in practice? What are the ethical, legal and regulatory issues we face globally in the application of AI?
Sotiris Bersimis, professor, elected member of the Board of Directors of the Hellenic Statistical Institute and elected member of the International Statistical Institute writes about the applications of AI in HR Management. Pavlos Avramopoulos examines important aspects of the European Union (EU) law regulating the use of Artificial Intelligence (AI Act). Specialist in AI, scientist, researcher and author, Giorgos Zarkadakis talks to us about the Past, Present and Future of Work and the impact of Artificial Intelligence on business and society, while Dr. Agathe Balayn, researcher at ServiceNow, at Delft University of Technology and at the University of Trento, with a PhD in Computer Science, talks about AI Debiasing and how organizations can address the issue of inclusion in the data that supply the models of AI systems.
We hope you enjoy and above all think creatively about everything that makes up the future of work, society and our collective coexistence and imagination.
EDITING TEAM FOR THIS ISSUE:
- Anastasia Makarigaki
- Dimitris Tzimas
- Mirela Dialeti
ARTWORK FOR THIS ISSUE:
DESIGN + WEB:
EXTERNAL CONTRIBUTORS:
- Sotiris Bersimis
- Pavlos Avramopoulos
- George Zarkadakis
- Agathe Balayn
LEGAL RESPONSIBLE:
- FurtherUp
- Skoufa 27, Athens
- info@furtherup-hr.com
- www.furtherup-hr.com
Applications of Artificial Intelligence in HR Management
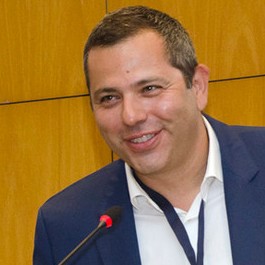
AI refers to the simulation of human intelligence by machines that are programmed to learn and make decisions like humans. AI systems can process massive amounts of data, learn from it, and make informed decisions in real time. In the heart of AI, one will find the methods, techniques and algorithms of the field of Statistical Machine Learning (SML).
SML is a toolbox of methods, techniques and algorithms that are installed in machines to "learn" from data. Specifically, SML focuses on the development of algorithms and models that can make predictions or predict the behavior of systems through the use of data. The SML, for its part, is based on methods of the science of Statistics. The learning process in SML simulates the way humans learn. Just as a person learns from observation and experiences, so does SML data in order to develop models and make predictions. Just as humans recognize patterns in data and draw conclusions, SML looks for patterns and correlations between data. The same way humans constantly learn from new data and adapt their knowledge, SML corrects and improves its models by adding new data. Humans can apply their knowledge to new problems they face. Equally, SML can be applied to new data or new problems to obtain or predict results. In conclusion, SML develops models that explain inferences from data, in a way that resembles the way humans learn.
In the area of HR
AI is gradually introducing a series of innovative applications that contribute, among others, to the improvement of the recruitment process, people management and employee development. Today, AI is gradually redefining the way it operates and processes in the field of human resources, and in particular the processes related to people management and employee development.
In relation to recruitment and candidate selection, AI can automatically read, analyze and rank thousands of resumes (as well as candidate characteristics on social networks), supporting HR managers in identifying the most suitable candidates for a position. In addition, the use of advanced AI systems that analyze real-time images and sound and can facilitate the interview process by providing initial assessments of candidates.
However, the use of advanced AI systems that make use of Genetic AI (Gen AI) can automate traditional tasks associated with the recruitment process, such as answering candidate questions, sending personal emails, etc.
In the context of selection, the concept of suitable candidate is a broad one. AI, for example, can, through the analysis of past data, classify candidates into categories according to the probability that they will remain for a long period of time in the organization. Long stay in the organization means minimizing the initial cost of onboarding and training the new executives of the organization.
In conclusion, AI significantly reduces both the time and the cost of the selection process while at the same time optimizing the selection of the most suitable candidates (suitable profile for the position).
In summary, AI in recruitment can mean
- Instant, automated collection of candidate data from multiple sources.
- Formation of an in-depth initial image of the candidates (profiling) by analyzing an extensive group of parameters of their CV, as well as image and audio data from the interview of the candidates.
- Identifying candidate characteristics that compare to the highest performing employees in the organization.
- Ensuring equal opportunities for all candidates as the assessment is data driven.
- Automation of the communication process with candidates.
In relation to people management
AI can analyze employee performance based on their data, providing personalized assessments and further improvements.
At the same time, predictive AI algorithms can predict the probability of an employee leaving the organization and recommend actions to retain him, if this is to the benefit of the business/organization. In addition, AI can provide forecasts of needs for organizations to formulate their strategic plans.
In summary, AI enables the people management process in
- Automated staff assessment.
- Predicting departures, which allows HR people to take proactive actions.
- Forecasting human resource needs, enabling organizations to formulate strategic and long-term staff development plans
In relation to employee development
AI can effectively identify training needs and offer tailored training programs, aiding in the continuous professional development of employees.
In addition, AI can propose personalized training programs for executives to learn, not only new skills, but also skills required in their position and in their daily operation. Also, AI can offer advice and guidance for personal development and career advancement of an individual through specialized recommendation systems.
In summary, AI during the employee development process enables can help with
- Identification of training needs and proposal of customized training seminars
- Suggesting personalized training programs for learning skills
- Advices and instructions for personal and professional development
By automating HR processes with AI, industry executives can significantly reduce their workload and improve their performance and efficiency, contributing to the growth and prosperity of businesses and organizations. Furthermore, with the extensive use of AI, an organization's HR executives and field professionals can focus on high-value strategic tasks that support the organizations' overall talent management, capability and employee development strategies.
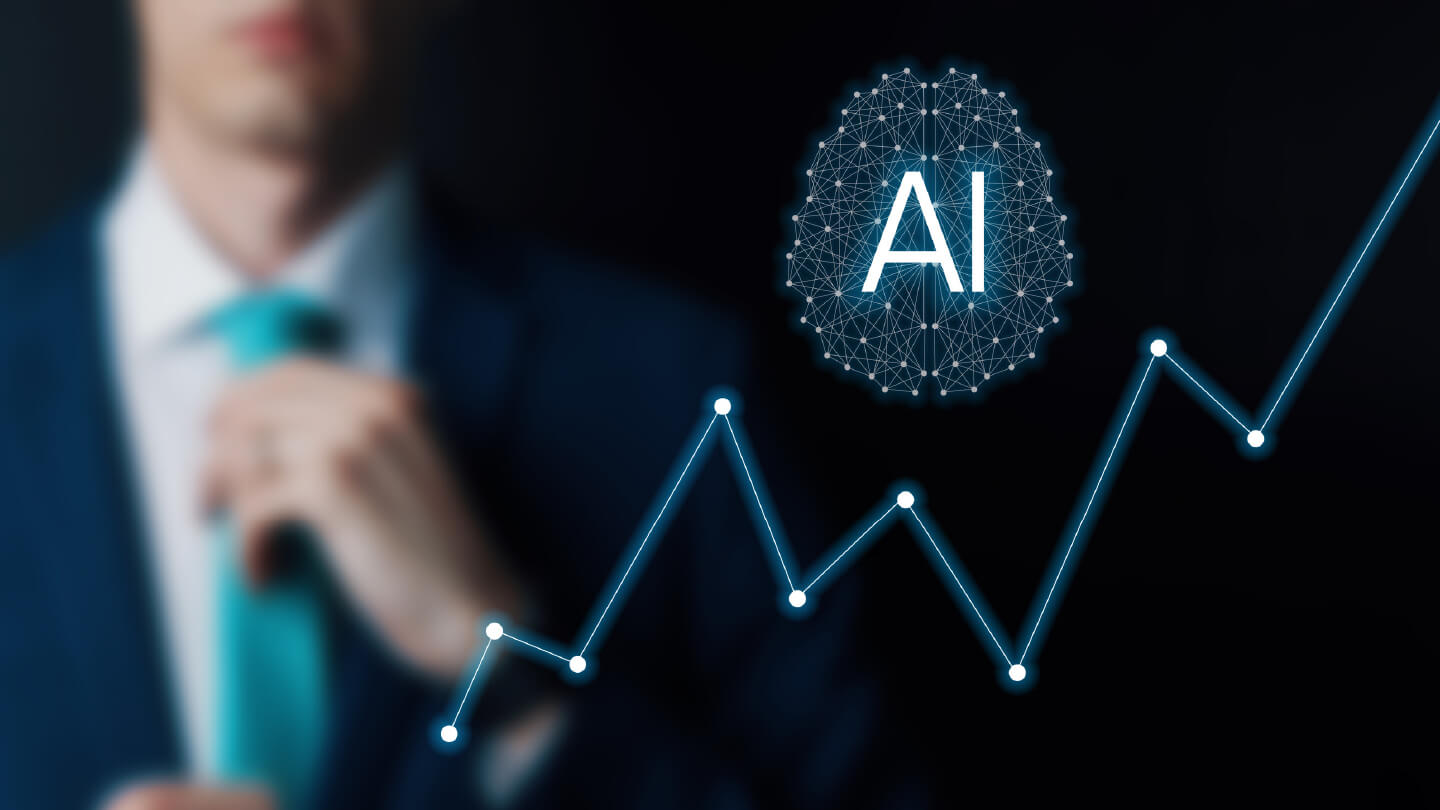
AI Evolution &
AI Revolution
A conversation with George Zarkadakis
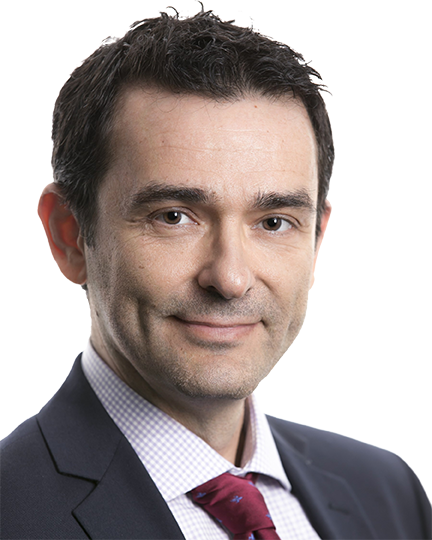
Mr Zarkadakis, you mention in your book “In Our Own Image, the History and Future of AI” many historical technological shifts in work due to the industrial revolution. Could you provide a brief overview of these shifts? How do you foresee AI influencing work dynamics in the contemporary work landscape?
Initially, prior to the first industrial revolution, people primarily engaged in agricultural and seasonal work, following natural rhythms that had persisted for thousands of years. However, with the advent of the industrial revolution, there was a significant shift in work patterns. This transformative period saw the emergence of a work model wherein humans became subordinate to machines: Over the past two centuries, human behavior has adapted around machines, with work hours aligning with electricity availability and tasks becoming increasingly standardized, often on production lines. This model, initially prevalent among factory workers, gradually extended to white-collar professions as well. As a result, our entire economy has become entrenched in a productivity-driven concept of work, characterized by mechanistic principles.
We are now entering a period of enormous shifts due to AI.
The first thing to say is that this technology is evolving by leaps and bounds. The biggest leap happened first in 2000, and then in 2020 there was a huge leap, a leap that no one expected to happen.
In 2000-2005 and right after, an approach to AI that was not the dominant one till then, created due to cheaper computational power and an increase in the availability of data, in connection with the development in some statistical models of machine learning, gave AI the form it has today, which is based on neural networks (Neural Networks) - in contrast to another form of AI that worked with what we call “symbolic logic”. Suddenly we have systems that can, for example, recognize faces. In 2017, a paper came out from a group at Google, that talked about a type of neural network architecture called “transformers”, upon which LLM-GENAI is based. This is a huge leap, because before that we had an artificial intelligence that was "narrow" i.e. each system had a specific application-now we have a general purpose intelligence system that behaves to us as we thought AI would behave to us in 20-30 years. This is evolving incredibly quickly. So, even due to speed, I don't think we can react with relative safety. Let's say regulators already tried to come up with an AI Act that was based on a view, perception and awareness of how AI works that could already be outdated. So the biggest impact of AI today is its speed.
Consider an example regarding intellectual property (IP). AI systems are trained using data from the world wide web. Suppose you're a writer, artist, or photographer who has authored a book. The AI can learn from your work, and if instructed, it can generate a follow-up piece that emulates your style and content, essentially writing on your behalf.
In economics, there's a phenomenon known as the "lump of labor fallacy." This misconception suggests that if someone takes a portion of available work, the remainder will persist. It's commonly believed that the amount of work accessible to individuals is fixed. However, this notion is flawed. Our economy demonstrates that new forms of labor continually emerge even as existing tasks are completed. AI systems, such as LLMs, exhibit emerging behaviors, including some capabilities for mathematical calculations despite lacking specific training in this area. As a result, new professions have arisen to study the behavior of these machines.
With the ever-evolving landscape of tasks, there's an increasing demand for upskilling to remain competitive and enhance productivity. As technology advances, the necessity for ongoing learning and skill development becomes even more critical in ensuring success in the workforce.
This highlights Microsoft's strategic positioning as a leading developer of AI technology, given its essential role in powering infrastructure worth tens of millions of dollars. The energy consumption of GPT Chat, for instance, is comparable to that of the entire nation of Greece, emphasizing the scale of operations involved. Recognizing the potential for robust artificial intelligence development, Silicon Valley invested heavily in data and computing power, resulting in the creation of advanced AI models like LLMs. These tech giants, including Microsoft, maintain a stronghold in this domain. Microsoft's "Co-pilot" initiative exemplifies this trend: as a white-collar worker, I now have an AI system at my disposal, significantly enhancing productivity. For example, with the assistance of an LLM, I could complete a PhD in one month instead of three years. The LLM would handle tasks such as information collection, reading, summarization, leaving me to direct the process efficiently.
Could AI systems free up, in a way, the employee from tasks that could take too much time and learning to do, and therefore let him develop more, let's say, soft skills such as communication or empathy? To be, for example, more creative, more collaborative?
We think that AI does not possess creative capabilities itself, but that’s not true, GEN AI actually does. Take, for instance, industries like cinema or theater, traditionally requiring collaboration among various professionals. With modern AI systems, it's conceivable that only a director would be necessary, rendering other roles redundant—this shift partly explains recent strikes in America*.
Similar to how the internet transformed print media, today's LLMs, versatile and imaginative, are reshaping the workforce landscape. This poses a threat to experts, as proficiency in crafting effective prompts becomes paramount; the better the question, the more insightful the LLM's response. While the skill of inquiry remains a distinctly human trait, AI, particularly Generative AI excels in generating answers.
Moreover, LLMs now exhibit proficiency in understanding social context, addressing a longstanding challenge in AI development—the integration of common sense—previously elusive until recent advancements.I don't believe there are any skills these models can't replace. The fundamental concept of work is undergoing a profound transformation, one for which many people are unprepared. Existing institutions must adapt to accommodate these shifts. History demonstrates how technological advancements precipitate significant social, economic, and other transformations. We say that these immense technological shifts will bring either evolution or revolution. Forward-thinking societies endeavor to proactively regulate and assimilate these changes, steering towards the evolutionary path.
*In May 2023, 11,500 members of the Writers Guild of America went on strike, demanding fair wages; better residuals, which have shriveled in the streaming era; and assurance that AI would not take their place as screenwriters in the future.
What kind of setskills can employees invest in, to prepare for the future of jobs in the era of AI?
Well, first of all, from an economic standpoint, immediate job loss isn't an issue for now. However, remuneration for these roles may diminish over time. Societies resistant to change, lacking in social adaptability, risk stagnation. It's evident that the challenge extends beyond individual readiness; societal preparedness is essential. Flexible societies that embrace change are better positioned to thrive. As a result, we may witness an influx of white-collar workers from nations where wage levels remain stagnant due to limited economic growth prospects. Numerous variables will influence the impact of these changes at the individual but also the economic, societal, and national levels. I think employees would be ready, first of all, for that.
So you say that just as societal fears may lead to retrenchment and closure for a society, an employee's perception of their future prospects influences their decisions on investment and direction. Their response to uncertainty and apprehension shapes their choices regarding where and how to invest in their future endeavors.
Certainly, it's not just individuals but also companies that will be profoundly affected by these changes. How companies respond to these dynamic changes will be crucial and will vary depending on factors such as their organizational structure, technological adoption, aggressiveness in seizing opportunities, philosophical point of view, and market strategies. Different sectors of the economy will experience varying impacts; for instance, the automation potential in software development could greatly benefit companies in that sector by streamlining processes. The internal restructuring of these companies to capitalize on such changes will be guided by management decisions.
How does the transformation from traditional large organizations to agile "micro-businesses" involve embracing technology stacks and cloud-native approaches to foster innovation and flexibility?*
This transformation is already underway, exemplified by the rise of individual contractors and freelancers utilizing platforms like Upwork and Fiverr.
These individuals are finding success due to various factors, including better alignment with their lifestyle expectations and the social dimensions of their work. Unlike the rigid model imposed by the industrial revolution, modern society craves flexibility in employment. The proliferation of platforms enables individuals to provide services without the need to establish full-fledged companies- the existence of which were primarily driven by the economic efficiency of consolidating labor under contracts, as outlined by the Theory of the Firm.
However, the emergence of platforms has streamlined this process significantly. Freelancers leveraging these platforms essentially operate as small businesses, collaborating with external partners and forming micro-companies. This dynamic contrasts with traditional organizational structures within companies. Transactions on these platforms prioritize skills over personal attributes or resumes. Work is disaggregating into activities that allow the implementation of talent-on-demand strategies. As a result, hiring decisions are based on the specific skills required rather than generalized qualifications. This shift in the hiring process is particularly significant amidst rapid changes, as companies may find themselves uncertain about their workforce needs.
So, we see that the integration of this evolving landscape into company structures has already begun. Internal platforms are being implemented within companies, allowing them to post jobs or projects for employees to join and collaborate on. This shift emphasizes a shift away from traditional processes inherited from the industrial revolution, towards a model focused on projects and platforms. The goal is to create an internal marketplace for skills, knowledge, and productivity, fostering motivation among employees to acquire the necessary skills to enhance their value within this dynamic environment. Central to this approach is the adoption of systems that facilitate navigation and empower employees to seek greater flexibility and satisfaction in their work.
*”How AI will make corporations more humane and super-linearly innovative”, Huffpost- Mr Zarkadakis talks about an AI transformational journey set to destroy the current rigidity of organizations and replace it with a network of fluid and agile “micro-businesses” collaborating across, and beyond, the organization.
How can HR leaders leverage AI and platforms to facilitate workforce transformation?
This transformation is integral to the digital transformation agenda.
HR plays a crucial role in supporting this shift by redefining performance management and measurement, incentivization methods, skill matching, and the review process. Unlike the annual review process inherited from the industrial revolution, today's approach is more dynamic and continuous. Despite the challenges of breaking away from established norms, particularly those rooted in the industrial revolution, history has shown that societies unwilling to adapt tend to stagnate and decline. This underscores the imperative for societies to embrace change in order to thrive—a fundamental principle of historical progression.
Furthermore, it is crucial for both unions and workers to not only accept but also actively guide this transformation for the betterment of all workers.
What is the current state of AI technology adoption in the Greek workforce, and what opportunities exist?
Well, I think Greece possesses significant potential and numerous advantages.
Think about it, we have a workforce equipped with new skills, strong higher education institutions, and well-developed infrastructure, including roads and internet connectivity. Greece also stands as a promising destination for companies looking to relocate some of their operations. I'm not familiar with what's happening right now, but I do think that Greece is poised for progress.
Links to Mr Zarkadakis’ recent work:
- His book on Democracy and Web3.0; “Cyber Republic” (MIT Press, 2020): https://mitpress.mit.edu/books/cyber-republic#preview
- Fortune oped on Data Trusts for UBI: https://fortune.com/2021/06/27/universal-basic-income-data-privacy-trusts/
- Atlantic Council blog post on decentralized identities for smart cities:
- https://www.atlanticcouncil.org/blogs/geotech-cues/how-to-secure-smart-cities-through-decentralized-digital-identities/
- Essay on web3, included in “Digital Humanism” book (Springer, 2022):
- https://link.springer.com/chapter/10.1007/978-3-030-86144-5_7
- TEDx talk on Citizen Assemblies: https://www.ted.com/talks/george_zarkadakis_reclaiming_democracy_through_citizen_assemblies
- Harvard Business Review article on Data Trusts https://hbr.org/2020/11/data-trusts-could-be-the-key-to-better-ai?ab=hero-subleft-2
- Huffington Post Column on AI and Society: https://www.huffpost.com/author/zarkadakis-252
- Harvard Business Review article on Future of Work: https://hbr.org/2016/10/the-3-ways-work-can-be-automated
Glossary of basic terms
Neural Networks: A neural network is a machine learning program, or model, that makes decisions in a manner similar to the human brain, using processes that mimic the way biological neurons work together to identify phenomena, weigh options and arrive at conclusions.
Symbolic Logic: Symbolic Artificial Intelligence (AI) is a subfield of AI that focuses on the processing and manipulation of symbols or concepts, rather than numerical data.
LLM: A large language model (LLM) is a language model notable for its ability to achieve general-purpose language generation and understanding. LLMs acquire these abilities by learning statistical relationships from text documents during a computationally intensive self-supervised and semi-supervised training process. LLMs are artificial neural networks, the largest and most capable of which are built with a transformer-based architecture.
Transformers based Architecture: Transformers are a type of neural network architecture that transforms or changes an input sequence into an output sequence. They do this by learning context and tracking relationships between sequence components. For example, consider this input sequence: "What is the color of the sky?" The transformer model uses an internal mathematical representation that identifies the relevancy and relationship between the words color, sky, and blue. It uses that knowledge to generate the output: "The sky is blue."
Gen AI: Generative AI (GenAI) is a type of Artificial Intelligence that can create a wide variety of data, such as images, videos, audio, text, and 3D models. It does this by learning patterns from existing data, then using this knowledge to generate new and unique outputs.
“Lump of Labor” Fallacy: In economics, the lump of labor fallacy is the misconception that there is a finite amount of work—a lump of labor—to be done within an economy which can be distributed to create more or fewer jobs. It was considered a fallacy in 1891 by economist David Frederick Schloss, who held that the amount of work is not fixed.
Theory Of The Firm: The theory of the firm refers to the microeconomic approach devised in neoclassical economics that every firm operates in order to make profits. Companies ascertain the price and demand of the product in the market, and make optimum allocation of resources for increasing their net profits.
Debiasing AI: On addressing and regulating AI inequalities
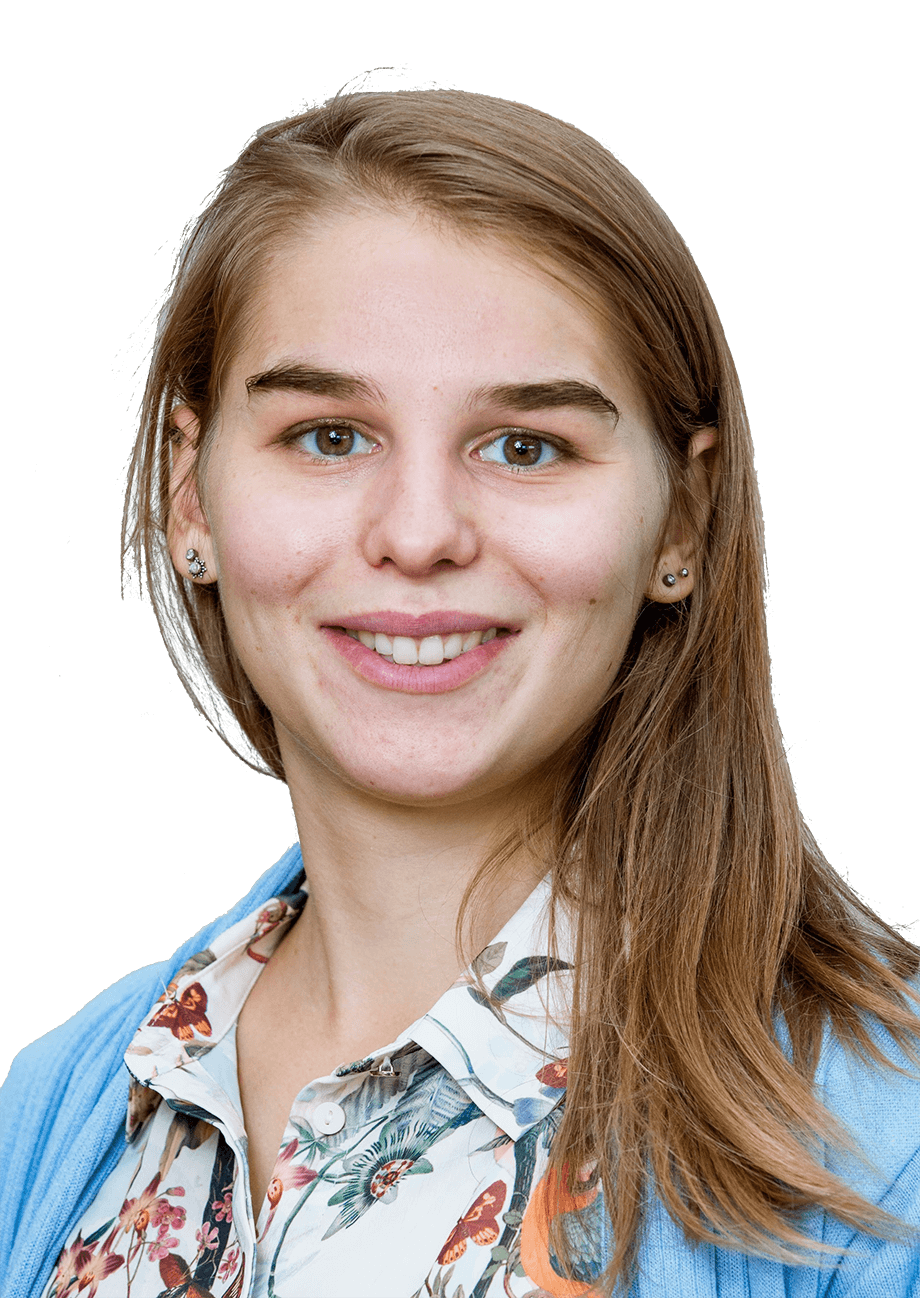
Agathe, can you tell us a little bit about how machine learning systems "learn" and how stereotypes are propagated through these machine learning modes?
Let's start by explaining first how we use machine learning tools: There are two primary stages, the first stage is training the model and the second is to use it in practice.
To train the model you need to collect data sets full of what you want the model to predict afterwards. For instance, in the context of HR, this could involve compiling CVs of both previously hired individuals and prospective candidates. These datasets serve as the foundation upon which the model will learn. So we have the datasets and then we choose the algorithms which are a set of equations with some parameters that have to be fixed. Then we do what we call “training of the algorithm” which is when you decide on the parameters, using the datasets that we prepared first.
The idea is that, hopefully, in the end, the parameters will represent the content of the datasets we have, so that later on, when using the algorithm with the parameters, it should be capable of making predictions based on new data inputs—such as determining whether a candidate is suitable for hire based on their CV.
I understand that you “feed” the algorithm with data. Therefore, if an individual with biases provides the algorithm with data, will the resulting system also be biased? Where does bias come from?
Typically, we begin with an algorithm containing certain parameters. As we optimize these parameters using the data, we transition to what we call “a model”. The model essentially represents the algorithm with the optimized parameters, derived from the data. Bias can manifest in various ways throughout this process.
One primary source of bias is the data itself used to optimize the parameters, which is collected and processed by individuals. For instance, if the CVs used to supply the dataset with information are predominantly from one demographic group, such as men, while other groups like women or people of color (POC) are underrepresented, the dataset becomes biased. Consequently, when optimizing the algorithm with this biased data, it may fail to effectively predict outcomes for underrepresented groups.
Additionally, the selection of the algorithm and the manner in which data is fed into it can also influence bias. Ultimately, it is the individuals developing the data, algorithm, and model who may inadvertently introduce bias due to their own predispositions or lack of awareness regarding the diversity or representativeness of the data.
In the final step, when creating the model, you require both the data and the fundamental algorithm, and the challenge lies in effectively integrating them. While the data itself can exhibit bias, as we saw, bias can also arise in the process of combining the data with the algorithm. There exist various approaches to this combination, each with its own implications for addressing or perpetuating biases present in the data. Some methods may inadvertently overlook certain biases in the data, while others may be more conscious of potential biases. However, it's important to recognize that even with awareness of one bias, there are mathematical constraints on what an algorithm can mitigate. For instance, while it may be feasible to mathematically address gender bias, tackling racial bias might pose additional challenges due to the intricate interplay of biases.
How do we currently address the discriminatory impact of AI?
So, because the bias can come from the data, from the algorithm and the way you combine the two, there are the main types of technical methods to debias the model. Some revolve around debiasing the data, some are based on the algorithm and the combination, and lastly, we can debias a model is the post processing of what the model does afterwards, after the creation of the model.
Debiasing the data involves increasing awareness of biases and taking proactive steps to address them. In the example we discussed, this might entail actively seeking out more CVs from women to balance the dataset. In computer science, a common approach is to synthesize new CVs, which involves duplicating existing ones and modifying them to reflect different genders or demographics. By creating synthetic data that closely resembles real-world examples, researchers can augment their dataset with more diverse samples, helping to mitigate biases and improve the overall fairness of the model.
When bias is inherent in the algorithm and its integration with the data, additional constraints can be imposed on the algorithm to ensure it prioritizes certain data points, such as CVs from women. This involves adjusting the parameters of the equation to emphasize the significance of women's data in the algorithm's decision-making process.
In the final step of debiasing the model through post-processing, the focus shifts to analyzing the outputs and the associated information provided. Typically, algorithms offer a level of certainty or confidence percentage for the decisions they make. When the certainty percentage is low, it may be prudent to involve human judgment in making the final decision. This step ensures that final human assessment is retained, especially in cases where the algorithm's confidence in its decision is compromised.
Before creating the model, it's crucial to establish its general purpose. For example, if the model is intended for CV acceptance or rejection, decisions must be made regarding the type of data to be used. However, obtaining unbiased data can be challenging, especially when attempting to include other non represented groups, let’s say non-binary individuals, in the process. Often, bias is unintentional, stemming from the limited availability of data and societal norms from the past.
An ethical dilemma arises in research areas where attempts are made to predict various characteristics, such as sexual orientation or criminal tendencies, based on biometric data and physical appearance. Such practices raise ethical concerns, as they may lead to unjustified predictions. It is now widely recognized that these characteristics are not significantly correlated with physiognomy or biometrics.
What are the implications of relying solely on debiasing algorithms and datasets to address discrimination in AI, and what alternative approaches could be considered?*
That's the challenge, in the end we don't have a good method to develop a model that is unbiased. Unbiased doesn't really mean anything in practice if you think about it, things are really subjective anyway, and that does mean bias. I've heard engineers tell me they are building unbiased GPT’S, removing all hate speech, but what constitutes bias can differ greatly depending on cultural context and legal standards. As a result, achieving complete unbiasedness in models is inherently difficult and subjective.
We do have metrics available to measure the level of bias in models, although they may not be entirely comprehensive. While these metrics may not provide a complete solution, they shouldn't be disregarded entirely as they can offer some insights. For example, in initiatives like the AI Act, efforts are being made to implement checks and balances. Having metrics allows us to ask important questions and serves as a starting point for addressing bias. The responsibility ultimately lies with developers and decision-makers though. They need to critically evaluate their approaches, question the diversity in the data, and consider how factors like gender are encoded. These decisions require thoughtful reflection and consideration, and while complex algorithms can be useful, they are not a substitute for conscientious design decisions.
Computer scientists often don't prioritize discussions about bias, as they may not consider it within the scope of their work. They see it more as an ethical issue and believe that decisions related to bias should be made by others, not them. In my interviews with many developers on this topic, I've found that a significant number of them work in organizations that lack the resources, time, and decision-making structures necessary for meaningful reflection on these issues.
*Given the limitations of debiasing techniques, policymakers should cease advocating debiasing as the sole response to discriminatory AI, instead promoting debiasing techniques only for the narrow applications for which they are suited- If AI is the problem, is debiasing the solution?
How can policymakers ensure that AI regulation protects, empowers, and holds accountable both organizations and public institutions as they adopt AI-based systems?
Currently, we lack specific measures to address bias in AI systems. While the EU AI Act outlines risk categories, translating these regulations into concrete bias checks presents a challenge. While computational researchers aim to create generalized methods, no one is willing to take sole responsibility for ensuring that these methods are free from bias.
The Act must also maintain a general framework, making it difficult to provide detailed guidance on bias mitigation. Developing standards tailored to various applications is essential, but computational scientists may not be adequately trained to deal with these complexities.
Do both decision-makers and computational scientists need education and awareness regarding bias in AI systems?
Certainly, because decision-makers should understand the importance of allocating resources and providing training for computational infrastructure, even if they don't fully grasp the technical aspects of data science. Computational scientists, on the other hand, should be aware of the impact of their decisions and may benefit from additional training or guidance on ethical considerations. In some cases, involving third-party companies with expertise in bias mitigation could provide valuable oversight and approval for decisions related to AI systems.
Do you think AI systems, being more “objective”, if regulated and used in the best way, could be allies of diversity, transparency and inclusion in business?
Well, the initial belief was that AI's objectivity could help mitigate human bias in decision-making processes. And the consistency of decisions made by AI models can indeed contribute to reducing bias compared to human decision-making, provided that the models themselves are carefully designed and trained to be fair and inclusive.
However, it's essential to ensure that AI systems are developed, deployed, and regulated in a manner that prioritizes ethical considerations, fairness, and transparency. This involves ongoing monitoring, auditing, and updating of AI models to address any biases that may arise and to ensure alignment with organizational values and societal norms regarding diversity and inclusion.
There is this rational worry that AI application reinforces computational infrastructures in the hands of Big Tech and that can influence the regulations around AI.
Of Course, lobbying efforts play a significant role in shaping the standards and requirements surrounding bias audits and regulations within the AI industry. Companies that provide AI models and infrastructure may exert influence over the definition of bias and impartiality based on their own values and social norms. This can result in specific biases being embedded into the algorithms and models they offer, reflecting the perspectives of their developers.
Furthermore, the power dynamics between organizations and AI providers can introduce complexities beyond just bias considerations. Organizations reliant on external AI infrastructure and models may face challenges related to decision-making autonomy, compliance with regulations like GDPR, and overall dependency on the technology provider. These dynamics underscore the importance of transparency, accountability, and ethical oversight in the AI industry to ensure fair and unbiased outcomes.
Also, the use of proprietary data by big tech companies to build AI models raises significant privacy concerns, particularly when these models are tailored for specific organizations. While these companies may have access to vast amounts of data from various sources, including their own platforms and their clients' data, questions arise regarding the handling and potential sharing of this sensitive information.
For instance, in the case of Large Language Models (LLMs), which require extensive data for training, the aggregation of data from multiple organizations poses risks of data leakage and breaches of confidentiality. Organizations may have legitimate concerns about the privacy and security of their data when it is used in such models, especially if they are unaware of how it will be utilized or shared.
Researchers are actively exploring methods to mitigate these privacy risks, such as techniques for data anonymization, encryption, and differential privacy.
How do you foresee the future in the use of AI tools for businesses?
I think we should take in consideration a lot of things, as beyond the concerns already highlighted, there are additional considerations surrounding the utilization of AI. The extensive data requirements necessitate significant computational power, posing environmental challenges. Moreover, the reliance on crowdworkers for data collection and annotation introduces ethical concerns regarding labor practices. These workers perform crucial tasks, yet their labor may be undervalued and exploitative. Therefore, it is imperative to address not only biases but also the broader ethical and environmental implications of AI deployment. This underscores the need for comprehensive regulation that addresses the diverse array of issues at hand.
In terms of solutions, personally, I would place more trust in a model created by a diverse team or a company that allocates resources and incentives for the development team to engage in reflection and thorough research prior to model creation. Additionally, when it comes to utilizing the model, it's crucial to consider whether biases are present and whether there are mechanisms in place for secondary human assessment or review to mitigate potential issues.
*Agathe especially talked about questions of bias/fairness in terms of gender, race, etc. But, she did emphasize that some scholars have also investigated the problems related to algorithmic hiring and disabilities https://dl.acm.org/doi/pdf/10.1145/3531146.3533169?casa_token=nK1BJ5PBSBUAAAAA:uz8Ujvc7IUSISZGG2XHSzg1Z0wny2oTAhtGOSMzXrd8D2IGyCoh-M1XNwxivQ2XCDXsnqqKeeOri
Glossary Of Basic Terms
Datasets: A data set (or dataset) is a collection of data.
Algorithm: In mathematics and computer science, an algorithm is a finite sequence of rigorous instructions, typically used to solve a class of specific problems or to perform a computation.Algorithms are used as specifications for performing calculations and data processing.
Parameters: Parameters in machine learning and deep learning are the values your learning algorithm can change independently as it learns and these values are affected by the choice of hyperparameters you provide. So you set the hyperparameters before training begins and the learning algorithm uses them to learn the parameters. Behind the training scene, parameters are continuously being updated and the final ones at the end of the training constitute your model.
Model: The final outcome after training of the algorithm, using parameters and datasets.
Synthetic Data: Synthetic data is information that's artificially generated rather than produced by real-world events. Typically created using algorithms, synthetic data can be deployed to validate mathematical models and to train machine learning models.
Encoding Gender: In computer technology, encoding is the process of applying a specific code, such as letters, symbols and numbers, to data for conversion into an equivalent cipher.
Crowd Workers: What we call crowdsourcing platforms are platforms where large, distributed groups of workers (named crowd workers) are given “microtasks” to help collect and annotate data intended for training or evaluating AI. On paper, it’s a clever solution to building the datasets quickly and easily given crowdsourcing’s ability to generate custom data on demand and at scale. However, it’s also an often overlooked if critical part of the AI lifecycle, in terms of ethical labor conditions.
ADMS: Automated Decision Making Systems are programmed algorithms that compute data in order to produce an informed automated decision on various matters. ADMS have been used in judicial, government and social services in order to remedy overworked and under-resourced sectors.
The new European Union law on artificial intelligence
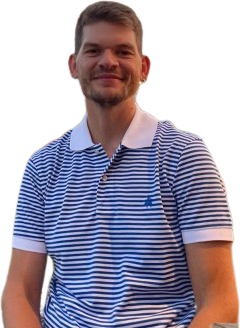
What is the EU Law on Artificial Intelligence?
The AI Act is a comprehensive legal framework proposed by the European Commission to regulate AI applications. It categorizes AI systems based on the risk to fundamental rights and security, ranging from 'high risk' to 'low risk'. The law aims to protect EU citizens from potential artificial intelligence gaps, while boosting innovation and competitiveness in the field of artificial intelligence.
Key aspects of the law include:
- Classification based on risk: AI systems are classified into four categories: Unacceptable risk, High risk, Limited risk and Minimal risk.
- Strict requirements for high-risk AI: High-risk AI systems, such as those used in critical infrastructure, law enforcement or employment, are subject to strict compliance requirements, including transparency, human oversight and strong data governance.
- Bans on certain AI practices: AI practices that are considered a clear threat to the rights and safety of individuals, such as social scoring by governments, are prohibited.
- Transparency obligations: AI systems that interact with humans, such as chatbots, must be designed so that users know they are interacting with an AI system.
Implications for businesses
- Businesses using or developing AI systems should invest in compliance infrastructure, particularly for high-risk applications. This includes implementing strong data management practices, ensuring transparency and maintaining human oversight.
- The AI Act could influence the direction of innovation by prioritizing the development of ethical, transparent and safe AI technologies. Compliance with the law could also be a market differentiator within and outside the EU.
- Failure to comply with the law can result in significant penalties, with fines of up to 6% of global annual turnover for the most serious violations. Businesses must be diligent in evaluating and classifying their AI systems to avoid legal implications.
- Companies may need to adjust their AI development and deployment strategies, focusing more on ethical issues and risk assessments from the design phase.
- As a pioneering legal framework, the AI Act is likely to influence global rules and standards for AI. Businesses operating internationally may need to consider the implications of the law beyond the EU.
Conclusion
The EU Law on Artificial Intelligence is a ground-breaking initiative in AI governance. It presents both challenges and opportunities for businesses. Following the law not only ensures compliance, but also encourages the development of AI systems that are ethical, transparent and beneficial to society. As the law shapes the landscape of AI use, businesses that proactively adapt to its standards will likely find themselves at a competitive advantage by leading responsible AI practices. The AI Act is not just a regulatory framework. It is a blueprint for the future of artificial intelligence in a society that prioritizes fundamental rights and security.
Τhe birth of the ΑΙ work environment
Progress in AI technology has transformed the HR department, enabling HR professionals to leverage machine learning and algorithms to streamline their work processes, reduce their biases, and enhance their analysis and decision-making.
This questionnaire will give us all* an indication of how fast HR people see the new AI dominated work environment approaching, as well as the type of issues that must be considered.
*We will share the results in our next newsletter issue together with any good practices.
(Please note that this questionnaire is impersonal and apart from the generic data, FurtherUp has no access to any personal data in relation to the respondents).
For more information on this you can contact our GDPR manager at info@furtherup-hr.com